Understanding A/B Testing
A/B testing, or split testing, involves comparing two versions of a webpage, email, or app to determine which performs better. This method helps businesses make data-driven decisions, enhance user experience, and optimize conversion rates.
Setting Clear Goals and Hypotheses
Before starting an A/B test, defining clear goals and hypotheses is crucial. Ask yourself:
- What do you want to achieve? You could increase click-through rates, improve sign-up rates, or boost sales.
- What is your hypothesis? Formulate a specific, testable hypothesis. For example, “Changing the call-to-action button color to red will increase click-through rates by 10%.”
Identifying Key Metrics
Determine the key metrics that will measure the success of your test. Common metrics include:
- Conversion Rate: The percentage of users who complete a desired action.
- Click-Through Rate (CTR): The percentage of users who click a specific link or button.
- Bounce Rate: The percentage of users who leave the site after viewing only one page.
Designing Your Test
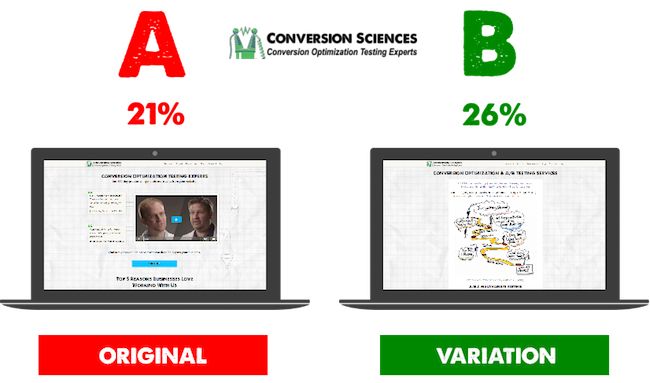
When designing your A/B test, it’s important to keep the following in mind.
- Control and Variation: The control is the original version, while the variation is the modified version. Ensure that only one element differs between the two to isolate the impact of that change.
- Sample Size: A statistically significant sample size is essential to ensure reliable results. Use online calculators to determine the required sample size based on your expected effect size and desired confidence level.
- Randomization: Randomly assign users to the control or variation group to avoid biases.
Running the Test
Implementing your A/B test effectively involves:
- Duration: Run the test for an appropriate duration to gather sufficient data. Ending a test too early can lead to inaccurate conclusions.
- Consistency: Ensure that users see the same version (control or variation) throughout their interaction to avoid confusion and maintain data integrity.
- Monitoring: Monitor the test to identify any technical issues or unexpected trends.
Analyzing Results
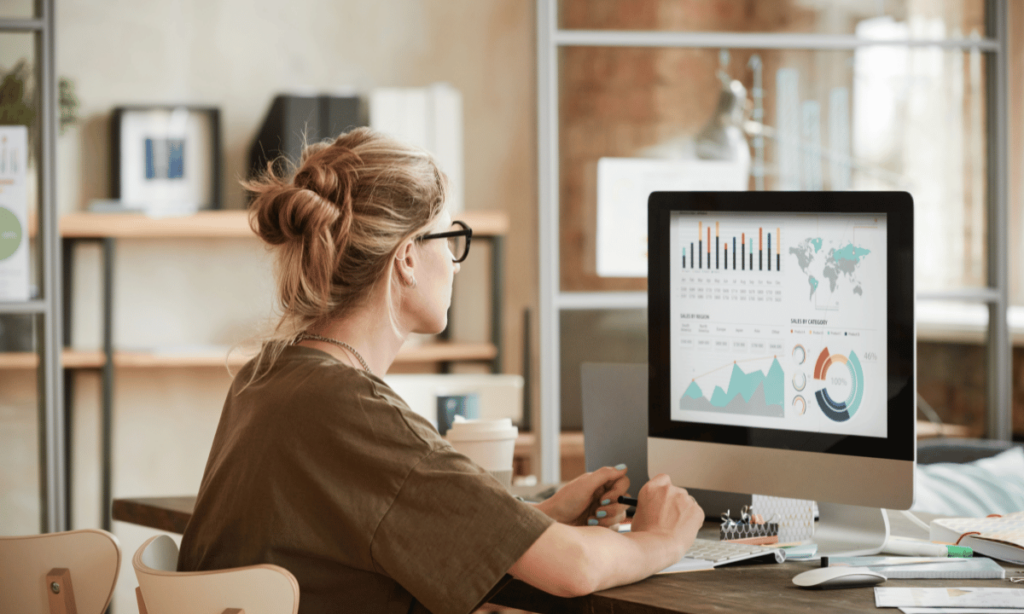
After completing the test, analyze the data thoroughly:
- Statistical Significance: Use statistical analysis to determine if the observed differences are significant and not due to random chance. Aim for a confidence level of at least 95%.
- Contextual Analysis: Consider external factors, such as marketing campaigns or seasonal trends, that might have influenced the results.
- Learning: Evaluate whether the test supports your hypothesis and what insights can be drawn from the results.
Implementing Changes
If the variation outperforms the control, implement the changes permanently. Even if the test doesn’t yield significant results, it still provides valuable insights into user behavior and preferences. However, it’s important to consider additional factors that may influence the outcomes.
Iterating and Continuously Testing
A/B testing is an ongoing process. Regularly test new ideas and improvements to optimize your digital properties continuously. Learn from each test and build upon your findings to drive sustained growth and improvement.
Common Pitfalls to Avoid
- Testing Multiple Elements: Changing multiple elements at once can obscure which change impacted the results.
- Insufficient Sample Size: Small sample sizes can lead to misleading conclusions.
- Short Test Duration: Ending tests prematurely can result in unreliable data.
- Ignoring Statistical Significance: Implementing changes without ensuring statistical significance can lead to ineffective or harmful modifications.
A/B testing is a powerful tool for optimizing user experience and conversion rates. Remember: “By setting clear goals, implementing effective tests, and accurately analyzing results, you can make data-driven decisions to enhance your digital marketing strategies.” Continuously iterating and testing new ideas will ensure ongoing improvement and success.